3D structured semantic scene graph
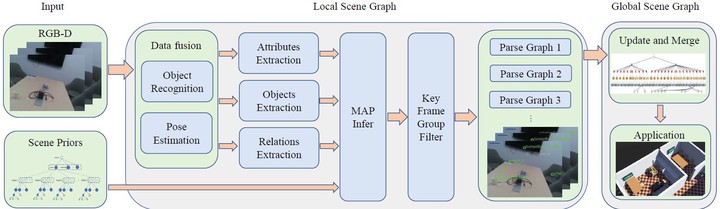
This paper presented a bottom-up framework of structured 3D scene graph generation from RGB-D image and scene priors. The proposed framework contains an improved grammar model, which is used to learn from scene dataset and describe the scene priors. The visual perception is used to capture the objects, relations and attributes from 3D scene, and the inference was adopted to obtain the optimal parse graph from scene priors. We implement our framework in a real indoor scene to demonstrate its accuracy in representing the semantic information, and the applicability of the high-level human-robot interaction navigation tasks is also verified in multi-room scene using human command. For further research, the dynamic semantic segmentation system can be used to improve the visual perception. The structure of the S-AOG is easy to expand, with the hierarchy of the S-AOG addition can help robots to execute more complex semantic tasks, such as autonomous exploration in unseen scene and the improvement of the visual perception using context grammar.
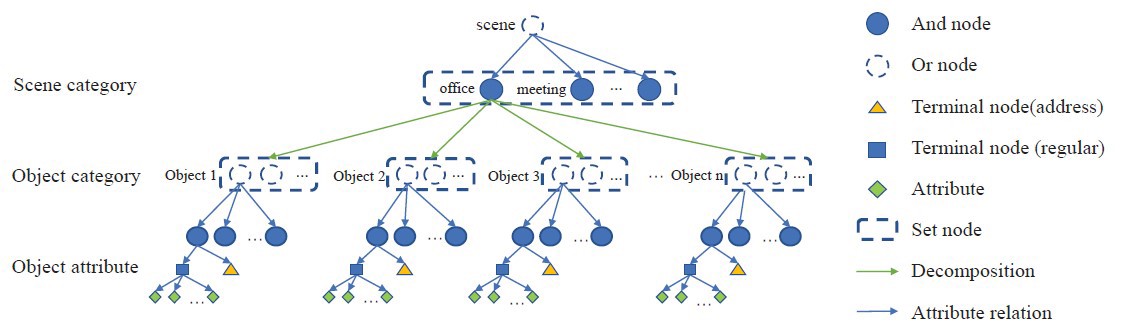
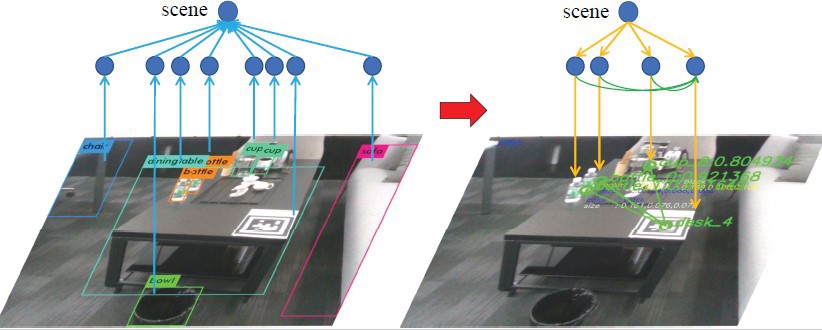
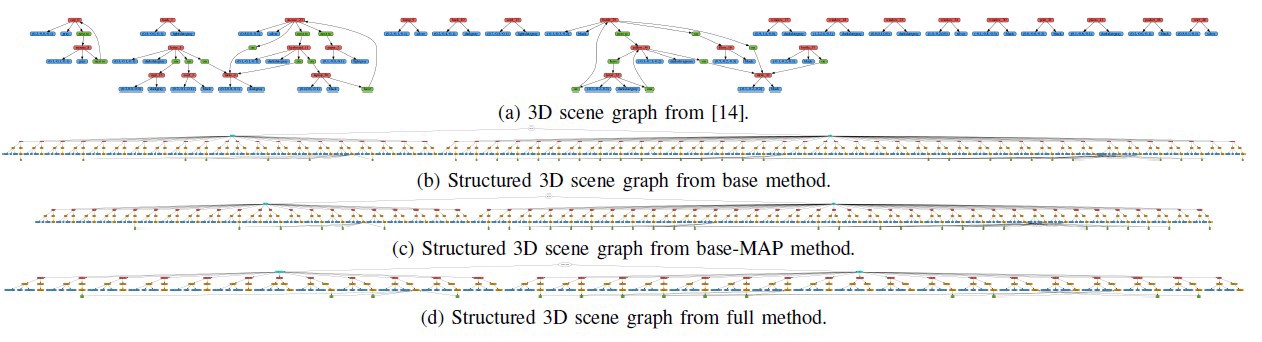
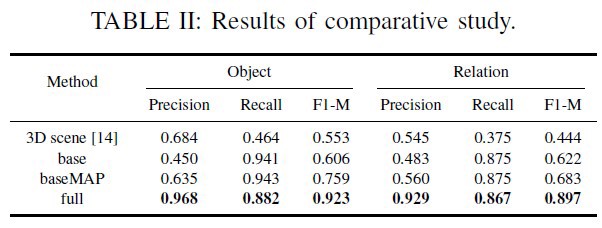
We use laser to provide the location information and use scene graph to achieve the target-driven navigation.
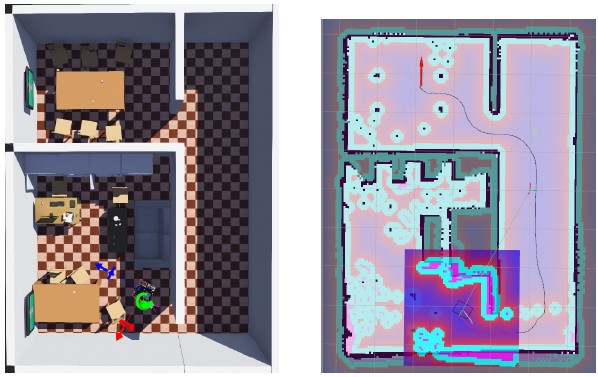